Case Study
Driving and defining change with expertise, compassion and persistence.
Case Study
Driving and defining change with expertise, compassion and persistence.
Case Study
Driving and defining change with expertise, compassion and persistence.
Customer Churn Prediction Model for Cryptocurrency Exchange
Customer Churn Prediction Model for Cryptocurrency Exchange
Customer Churn Prediction Model for Cryptocurrency Exchange
Background
A premier crypto exchange in Asia seeks to understand and reduce customer churn.
Problem Statement: Identifying customers at risk of churn to enable targeted retention strategies.
Address the challenge of customer retention in a growing user base.
A premier crypto exchange in Asia seeks to understand and reduce customer churn.
Problem Statement: Identifying customers at risk of churn to enable targeted retention strategies.
Address the challenge of customer retention in a growing user base.
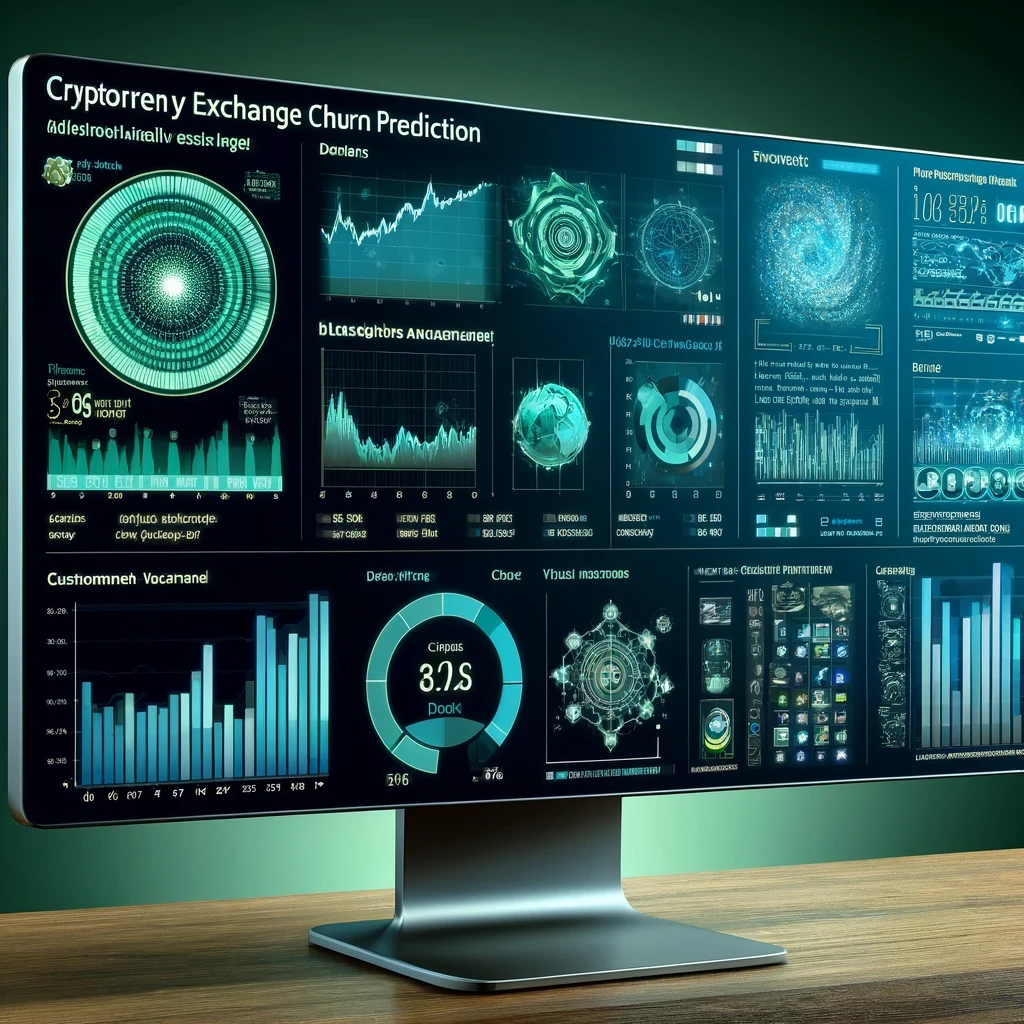
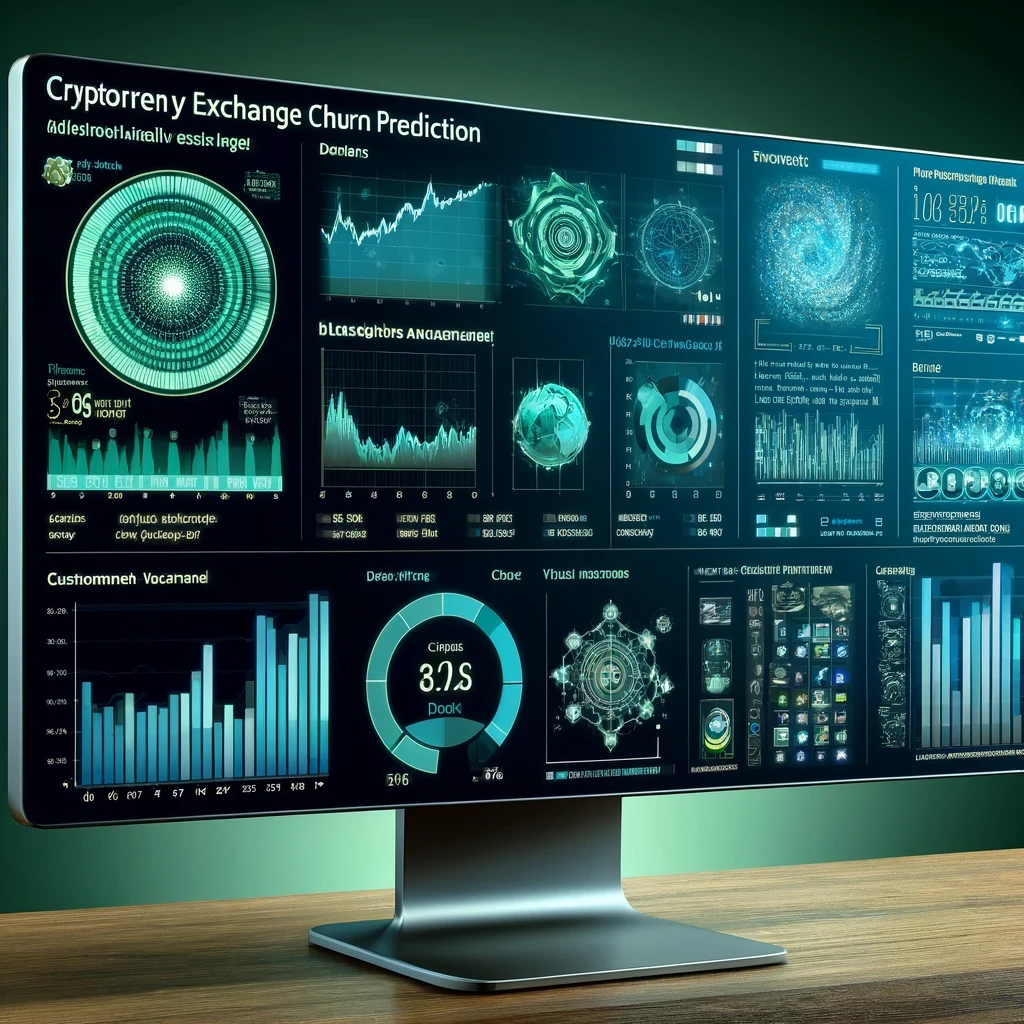
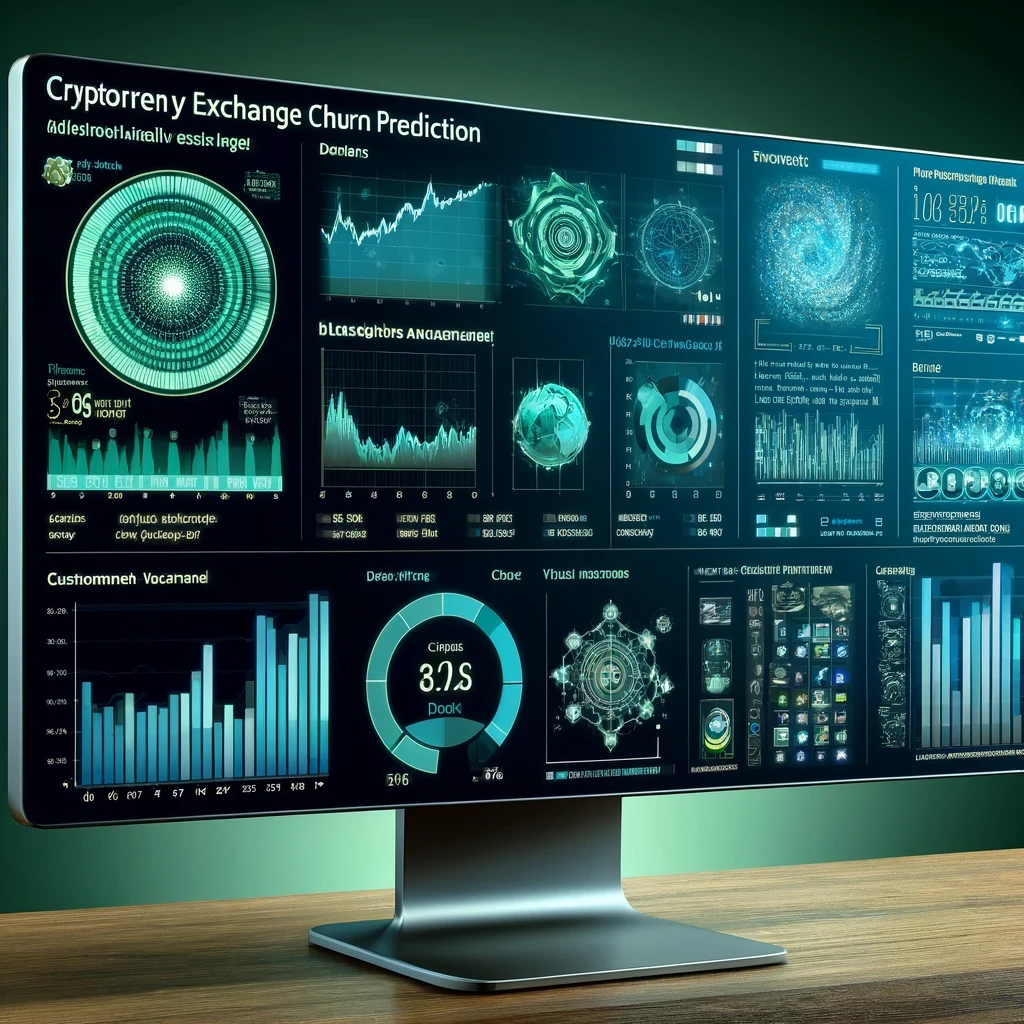
Design and Methodology
Mined crypto exchange’s private databases to collate 10 critical data features using SQL.
Mined crypto exchange’s private databases to collate 10 critical data features using SQL.
Mined crypto exchange’s private databases to collate 10 critical data features using SQL.
Distinguished between static personal details and dynamic trading behaviors.
Distinguished between static personal details and dynamic trading behaviors.
Distinguished between static personal details and dynamic trading behaviors.
Objective
Anticipate monthly customer churn
Anticipate monthly customer churn
Anticipate monthly customer churn
Model Development
Logistic Regression: Initiated modeling with a commendable 73% accuracy.
Logistic Regression: Initiated modeling with a commendable 73% accuracy.
Logistic Regression: Initiated modeling with a commendable 73% accuracy.
Random Forest: Refined to prevent overfitting by limiting tree depth, enhancing model reliability
Random Forest: Refined to prevent overfitting by limiting tree depth, enhancing model reliability
Random Forest: Refined to prevent overfitting by limiting tree depth, enhancing model reliability
XGBoost: Surpassed other models, demonstrating 82% precision and 91% recall using AUC as the benchmark.
XGBoost: Surpassed other models, demonstrating 82% precision and 91% recall using AUC as the benchmark.
XGBoost: Surpassed other models, demonstrating 82% precision and 91% recall using AUC as the benchmark.
Result & Impact
Actionable Insight
Delivered actionable insights to predict and mitigate churn, supporting customer retention and strategic business objectives.
Implementation into Operating Model
Operationalized model to the exchange’s marketing efforts.
© 2024 Gaialabs. All rights reserved.
© 2024 Gaialabs. All rights reserved.
© 2024 Gaialabs. All rights reserved.